[AI Day Spring 2024] Multi-modality, Enhanced LLMs, and Connect Workflows >>
Watch the RecordingDiscover LILT's research in Contextual AI
The LILT research team is driving the future of translation technology.
LILT's large language models are fine-tuned in real-time, as translators work, updating parameters automatically with each sentence translated. This tight loop allows adaptation to specific document-level and project-level vocabulary, structural patterns, and idiosyncrasies. Our research team focuses on the fast and effective contextualization of state-of-the-art neural machine translation models using methods that are efficient enough to support large-scale, personalized neural machine translation.
Contextual AI for enterprise translation doesn’t just enable the translation of full sentences in isolation; it must make suggestions with deep business and content context — predicting what translators will type next, how they will transfer formatting from the source document to the target, and what edits will be performed by reviewers. Truly interactive systems must take termbases, translation memories, and contextual constraints into account for all of these suggestions. Our research team focuses on the full range of automatically generated suggestions that can improve the speed and quality of human localization work across translation, reviewing, and quality assurance.
LILT's unique approach to localization places both professional translators and artificial intelligence technology together at the core of our operations. A broad range of human-computer interaction problems arise in this setting, from text-editing interfaces to assigning translators to project workflows. Our research team focuses on interaction design across the LILT platform and data science across LILT's business and translator community.
Meet the LILT Research Team
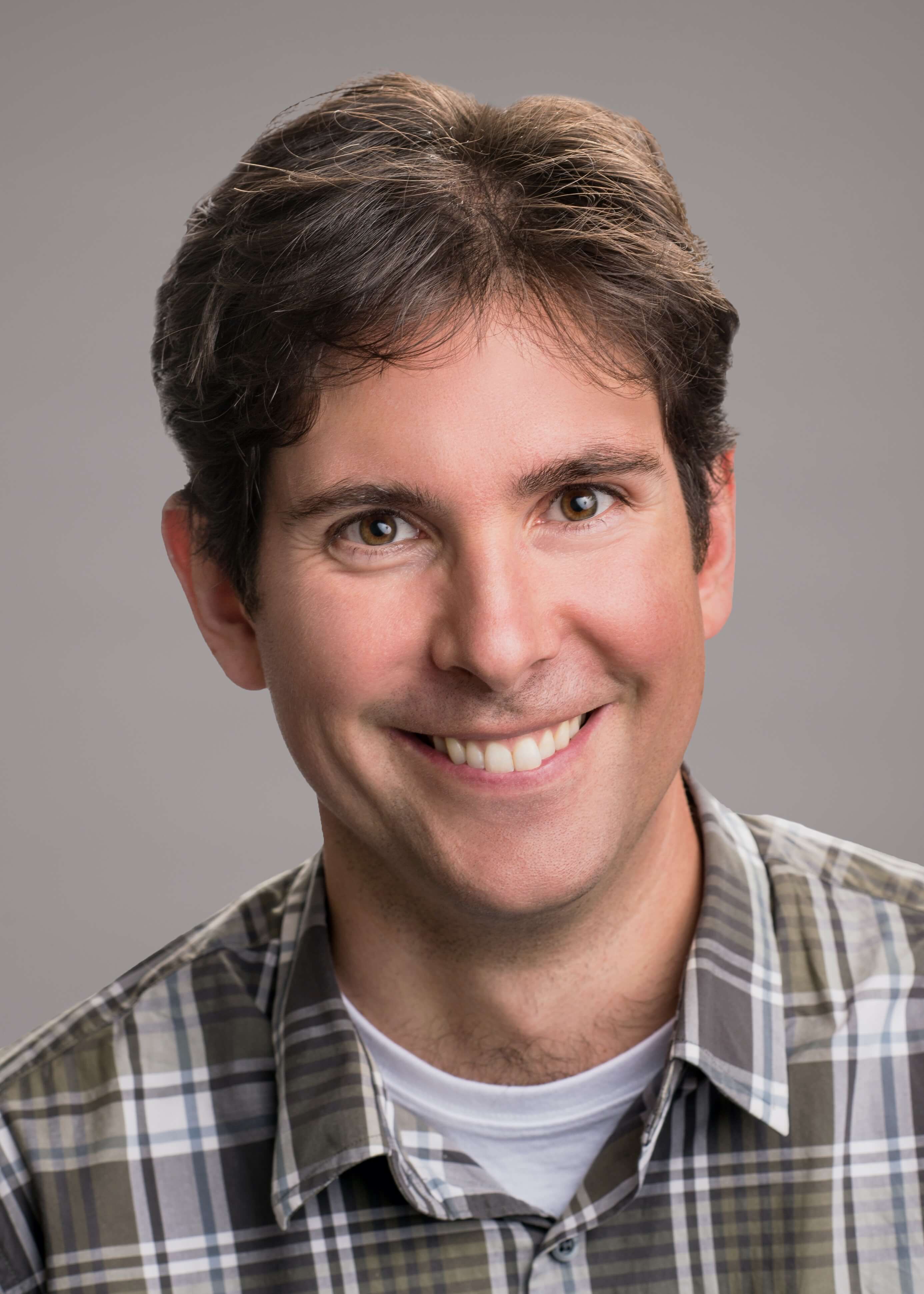
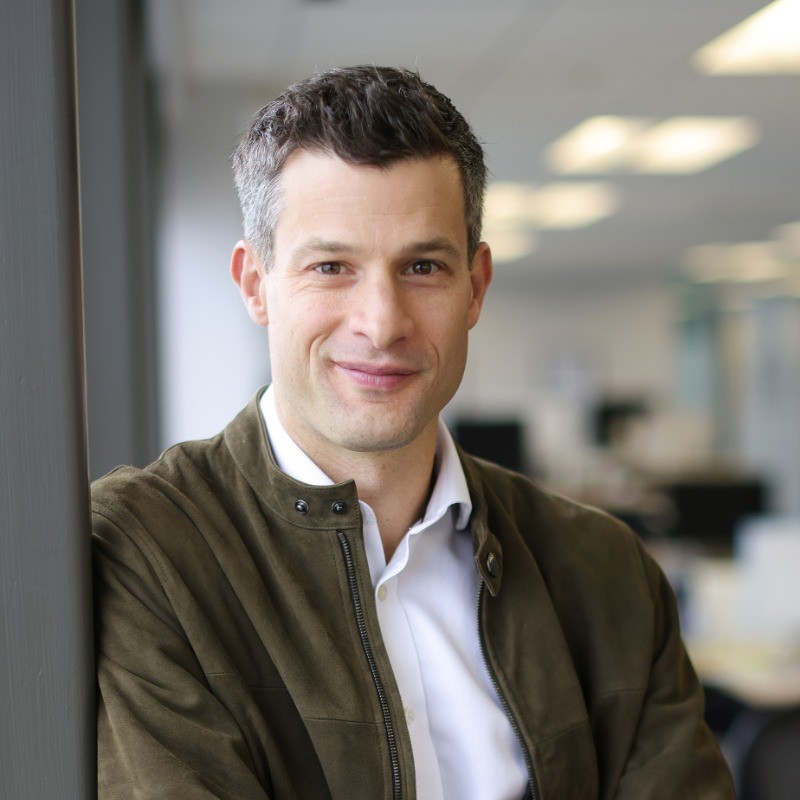
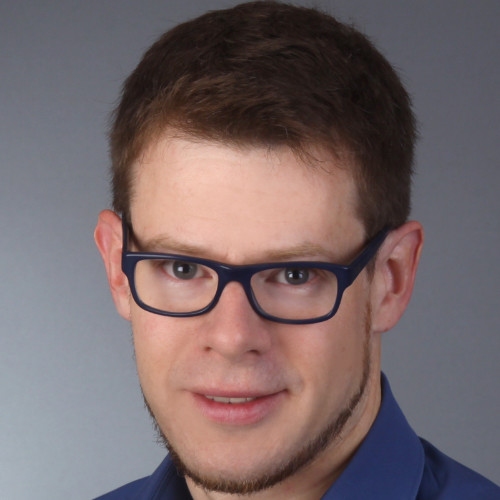
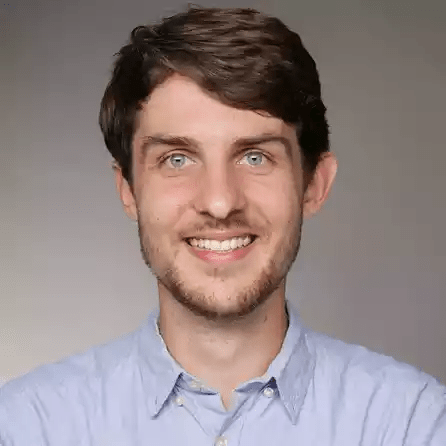
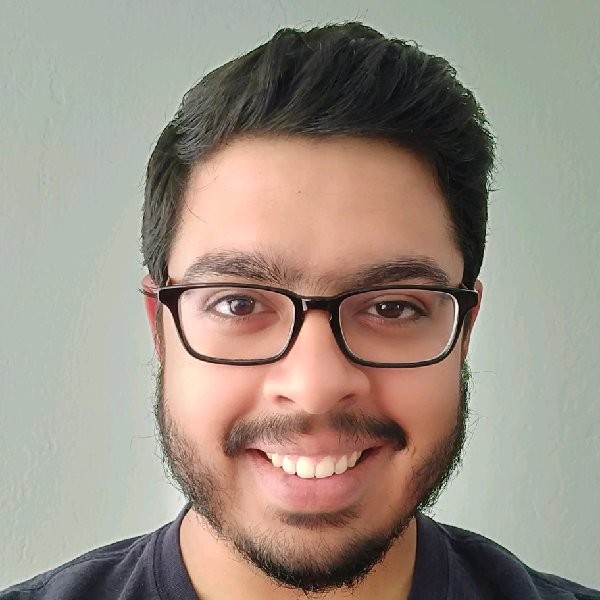
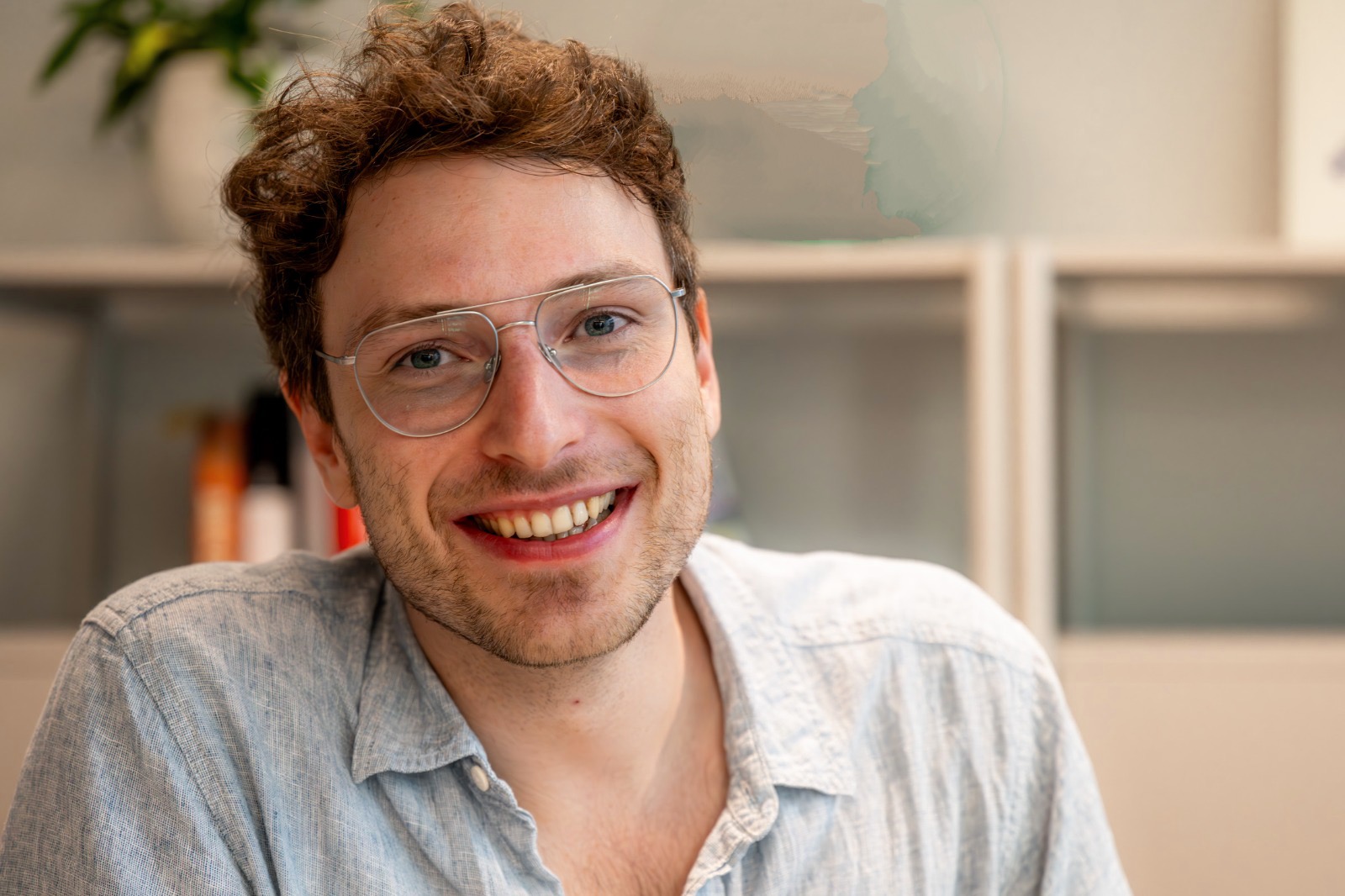
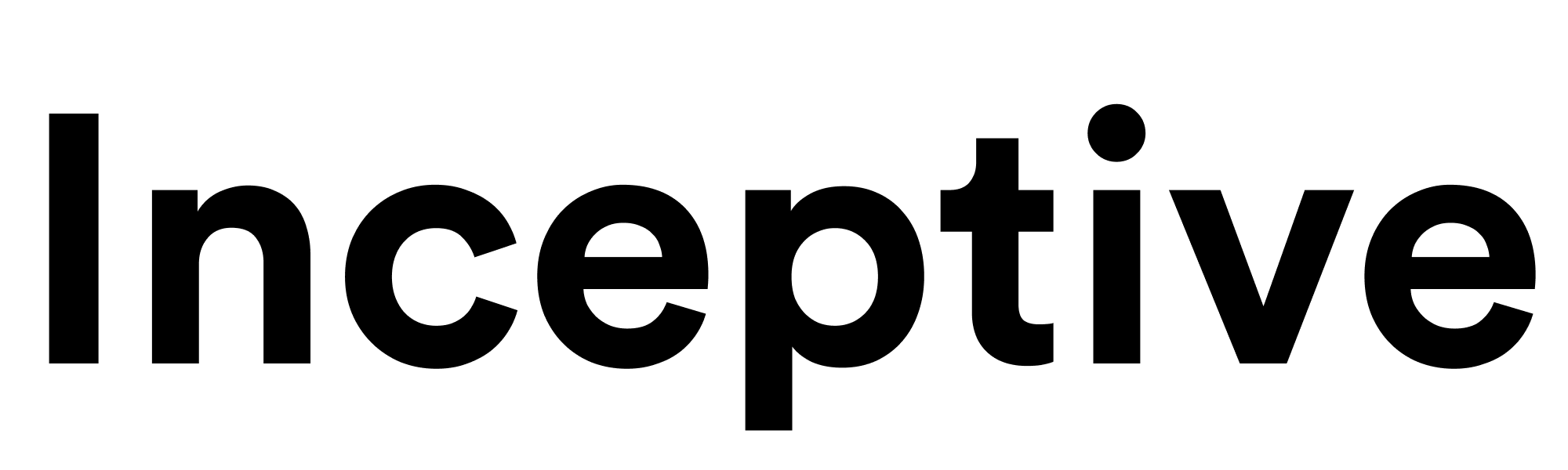
"So many interesting translation problems touch on cultural issues and contextual clues. If we really want to reach what we would consider the highest possible level of quality achievable, we have to build technology that better understands all people and cultures."
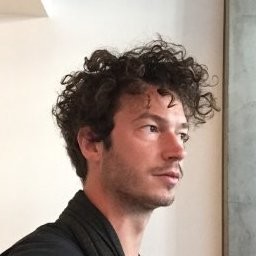