Overview: General Purpose LLMs vs Purpose-Built LLMs vs Custom LLMs
by
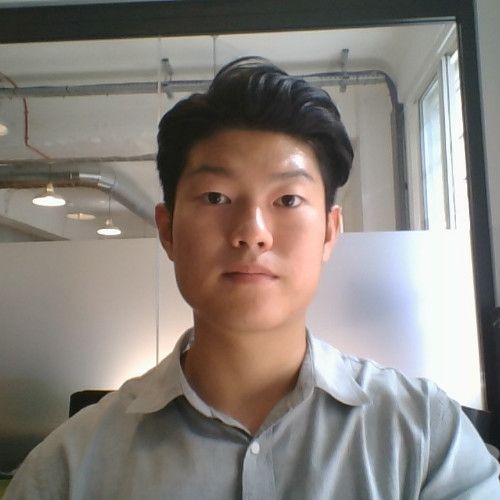
Lucas Kim, Content Marketing Manager
·AI
As enterprises increasingly leverage Artificial Intelligence (AI) to enhance operations, understanding the different types of Large Language Models (LLMs) becomes crucial. LLMs are the backbone of AI-driven language tasks, from content generation to translation. However, not all LLMs are created equal. They can be categorized into three main types: General-Purpose LLMs, Purpose-Built LLMs, and Custom LLMs. Each serves distinct needs, offering varying degrees of specialization and flexibility. This blog provides an overview of these LLMs, their key characteristics, use cases, and how they compare to one another.
Key Characteristics
General-Purpose LLMs:
- General-purpose LLMs, such as GPT-4, are pre-trained on vast and diverse datasets. These models are designed to handle a wide range of tasks, from text generation to question answering, without requiring further training or customization.
- Their versatility lies in their broad training data, which includes text from the internet, books, articles, and more. This makes them adaptable to various applications across different industries.
- While they excel in general tasks, they might lack the specificity needed for highly specialized domains.
Purpose-Built LLMs:
- Purpose-built LLMs are designed for specific industries or tasks. They are trained on domain-specific data, making them particularly effective in specialized fields like legal, medical, or financial sectors.
- A standout example is LILT’s contextual AI, which offers real-time adaptation and model customization directly from the translator interface. This allows the model to continuously adapt and learn from human-verified data, ensuring high accuracy and relevance in translations.
- Purpose-built LLMs are highly accurate in their respective domains but may not perform as well outside their specialized area.
Custom LLMs:
- Custom LLMs are tailored models that begin with a general-purpose LLM and are fine-tuned with proprietary or domain-specific data provided by an organization.
- These models offer the flexibility of general-purpose LLMs combined with the precision of purpose-built models. For platforms like LILT, custom models are integrated with their general-purpose LLMs, enabling the creation of highly effective autonomous systems.
- Custom LLMs are ideal for organizations with unique requirements, allowing them to align the model’s output with their specific business needs.
Use Cases
General-Purpose LLMs:
- Suitable for a broad array of applications, including content creation, customer service automation, and basic translation tasks.
- Used in environments where a versatile, all-purpose language model is needed to handle various tasks without the need for specialized knowledge.
Purpose-Built LLMs:
- Ideal for industries that require domain-specific expertise. For example, in healthcare, a purpose-built LLM trained on medical texts can generate accurate medical reports or provide clinical decision support.
- In legal settings, a purpose-built LLM can analyze contracts and legal documents with a high degree of accuracy, thanks to its specialized training data.
Custom LLMs:
- Perfect for organizations that need a model customized to their specific needs. For instance, a global retailer might fine-tune a general-purpose LLM with data related to their products and customer interactions, creating a model that can generate highly relevant product descriptions or personalized marketing content.
- LILT Create is a prime example of how custom LLMs are utilized within the LILT platform, enabling users to instantly generate brand-aligned multilingual content.
Comparison
Versatility vs. Specialization:
- General-purpose LLMs offer broad applicability, making them suitable for a wide range of tasks. However, they might lack the depth needed for specialized tasks.
- Purpose-built LLMs excel in their specific domains, providing high accuracy and relevance but limited versatility.
- Custom LLMs strike a balance, combining the broad capabilities of general-purpose models with the specialized accuracy of purpose-built LLMs.
Training and Data:
- General-purpose LLMs rely on large, diverse datasets to provide versatility.
- Purpose-built LLMs are trained on curated, domain-specific data to ensure precision in niche areas.
- Custom LLMs start with general-purpose models and are fine-tuned with proprietary data, making them highly tailored to specific organizational needs.
Integration and Flexibility:
- General-purpose LLMs can be easily integrated into various applications but may require additional customization for specialized tasks.
- Purpose-built LLMs are less flexible but provide superior performance in their targeted domains.
- Custom LLMs offer the best of both worlds, providing tailored solutions that meet specific business needs while maintaining the versatility of general-purpose models.
Conclusion
To build effective AI-driven systems across multiple business units at scale, enterprises need to leverage all three types of LLMs—general-purpose, purpose-built, and custom. Each plays a crucial role in creating a comprehensive AI strategy that meets the diverse needs of a global organization. Purpose-built LLMs are essential for training general-purpose LLMs, ensuring that custom LLMs are built with high-quality, verified domain-specific data. Moreover, working with interoperable systems is crucial to seamlessly integrate these models, ensuring that the AI solutions are robust, adaptive, and capable of driving success across the organization. By integrating these models and harnessing their unique strengths, businesses can transform their operations, driving innovation and efficiency in the age of AI.