How LILT Helps Foundational AI Models Become Multilingual
by
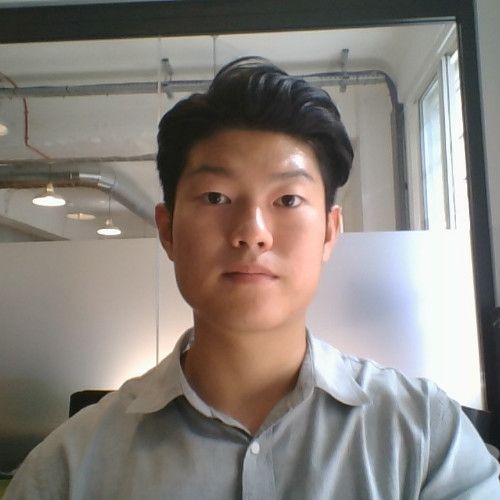
Lucas Kim, Content Marketing Manager
·Data Labelling
Language should never be a barrier. Yet for all their remarkable capabilities, even the most advanced AI models struggle with a critical shortcoming: they're predominantly built on English data in an overwhelmingly multilingual world. This disconnect creates a significant performance gap that limits the global potential of AI technologies and reinforces linguistic inequalities in digital spaces.
Consider this striking reality: while English dominates 43% of web content, only 5% of the global population speaks it as a first language. With over 7,000 languages spoken worldwide, most leading AI models are trained on roughly 100 of them—leaving vast linguistic territories unexplored and underserved. High-quality data labelling across diverse languages has become the missing piece in truly universal AI.
For enterprises pushing the boundaries of AI innovation, this isn't just a philosophical problem—it's a competitive limitation with real-world consequences.
The Foundation of Natural Language AI
The development of AI language models has been marked by continuous efforts to make them sound more natural and human-like. A significant breakthrough came with Reinforcement Learning from Human Feedback (RLHF), a technique that revolutionized how AI models learn to communicate. RLHF works through a sophisticated process where human evaluators rate the quality of AI-generated responses, creating a reward signal that helps the model learn which responses are more natural and appropriate. This process begins with initial training on vast text datasets, followed by generating multiple response variations, gathering human feedback on response quality, and finally fine-tuning based on this collected feedback. While this approach has proven highly effective for English-language development, achieving the same level of naturalistic output in other languages presents a significant challenge.
The Multilingual Challenge in Modern AI
The performance disparity between English and non-English language capabilities in today's AI models reveals a substantial and systemic gap. Even advanced AI systems show noticeable degradation when generating content in languages beyond English, manifesting in misinterpretations of cultural context, grammatical inconsistencies, stylistic awkwardness, and limited idiomatic expression. Organizations working to develop truly multilingual AI face interconnected obstacles, including the scarcity of high-quality training data in non-English languages, complex operational requirements for multilingual evaluation, the necessity for deep cultural understanding beyond literal translation, and maintaining consistent quality control across diverse language systems.
LILT Human Eval: Pioneering Global AI Communication
LILT Human Eval emerges as a specialized solution for these challenges, offering a systematic approach to improving AI language capabilities across multiple languages. The platform leverages native speakers to help AI models develop more natural, culturally appropriate responses in their respective languages. This comprehensive system combines an AI-native platform specifically designed for multilingual evaluation, expert AI research capabilities, and a global network of verified native-speaking experts. As AI increasingly powers global applications, the ability to communicate naturally in multiple languages has become crucial. LILT's Human Eval system helps bridge this gap by enabling AI models to develop more authentic, culturally appropriate communication styles across different languages and regions.
Image (Updated Screenshot)
Key Features & Benefits
Multilingual human eval stands apart through several core capabilities that directly address the challenges of multilingual AI development:
Enterprise-Scale AI Platform. At the foundation of Multilingual human eval is a sophisticated SaaS platform specifically engineered for managing multilingual data labelling and evaluation at scale. Unlike general-purpose annotation tools, LILT's platform incorporates specialized features for multilingual text handling, including segment-by-segment translation, research tools for challenging phrases, and seamless integration of style guides and instructions. The platform's intuitive workflow management capabilities streamline the entire evaluation process—from prompt creation and file uploading to reviewer assignment and quality assurance. This infrastructure enables organizations to execute large-scale data labelling projects without the operational overhead typically associated with multilingual initiatives.
Deep AI Research Expertise. Raw technology alone isn't enough to solve complex multilingual challenges. LILT brings over a decade of specialized experience in building and optimizing translation models, with an AI research team that has produced numerous scientific publications in the field. This expertise manifests in everything from evaluation methodology design to data labelling frameworks optimized for multilingual contexts. When technical challenges arise—as they inevitably do in cutting-edge AI work—LILT's researchers provide both practical solutions and strategic guidance that accelerates progress.
Global Domain Experts. Perhaps most critically, LILT has built a rigorously verified community of over 5,000 native-speaking experts spanning more than 40 specialized domains. Each expert is selected according to ISO-17100 standards, ensuring both linguistic proficiency and domain knowledge that captures the cultural nuances essential for accurate data labelling. This global community enables consistent coverage across more than 100 languages, with dedicated talent management ensuring continuous quality and responsiveness. Rather than the generic crowdsourcing approaches common among competitors, LILT maintains a carefully curated expert network with specialized skills that match the specific requirements of AI evaluation tasks.
Quality Assurance & Fraud Prevention. Quality concerns often plague human evaluation efforts, particularly in multilingual contexts where oversight becomes exponentially more complex. LILT addresses this challenge through built-in observability systems that monitor every annotator working session in real-time, automatically flagging potential quality issues and detecting unauthorized use of machine translation tools. This proactive approach to quality assurance, combined with ISO-certified processes and enterprise-grade security (SOC2 and Cyber Essentials certified), ensures that evaluation data consistently meets the highest standards—a critical requirement for model improvement efforts where data labelling quality directly impacts outcomes.
Cost Efficiency. Despite these premium capabilities, LILT delivers evaluation services at per-word prices up to 50% lower than legacy data labelling providers. This cost efficiency stems from purpose-built technology that automates routine aspects of the evaluation process while focusing human expertise where it adds the most value.
Target Applications & Use Cases
Multilingual human eval supports the full spectrum of multilingual model improvement needs:
Comprehensive Model Evaluation. Benchmark model performance across languages through systematic assessment of outputs, measuring key factors like factuality, context relevance, completeness, and potential biases. This creates a reliable foundation for targeted improvement efforts.
Supervised Fine-Tuning. Generate high-quality prompt/response pairs from domain experts in target languages, create custom prompts for specific use cases, and develop expert responses that capture linguistic nuances missing from standard training data.
Reinforcement Learning from Human Feedback (RLHF). Collect nuanced human feedback on model outputs from native-speaking experts, enabling the development of sophisticated reward models that guide model optimization across different languages and domains.
Data Curation & Expansion. Evaluate existing multilingual datasets for quality and relevance, expand coverage into underrepresented languages, and ensure cultural appropriateness across diverse contexts through specialized data labelling techniques.
The impact of these capabilities is transformative. As one Fortune 10 model provider reported: "We saw amazing improvements in our models for Bulgarian, Swedish, Hebrew, Indonesian, and Dutch based on datasets provided by LILT."
Building Better Multilingual AI
As AI continues its rapid evolution, multilingual performance will increasingly separate market-leading models from the rest of the field. Organizations that systematically address their models' linguistic limitations through comprehensive data labelling now will gain significant advantages in global markets and diverse user communities.
LILT Human Eval offers a proven path to multilingual excellence—combining specialized technology, world-class expertise, and global scale to close the performance gap between English and non-English capabilities.
Ready to transform your model's multilingual performance? Learn how LILT's human evaluation and data labelling solutions can accelerate your progress.